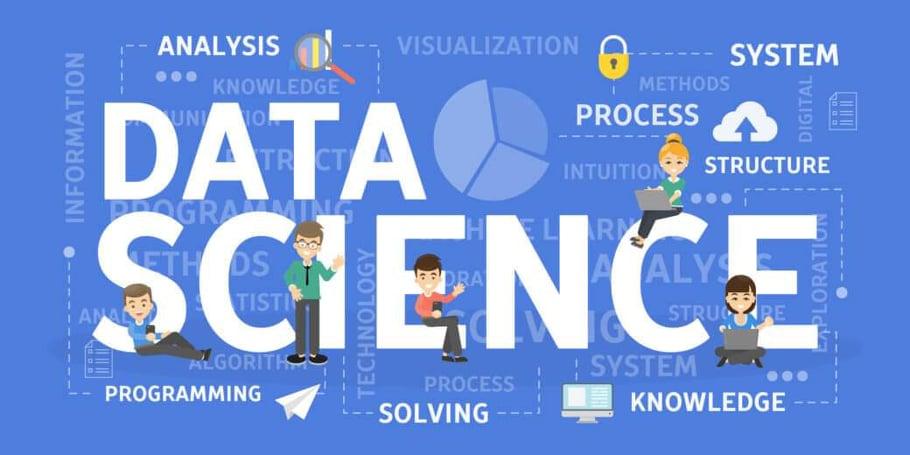
I recently had the opportunity to meet with Dr. Shahzad Cheema, a major data scientist at IBM IOT Industrial Laboratory in Munich. We have interesting discussions about data science and its applications in the real world. Dr. Cheema, the data science course may be the most interesting and least understood field in it. Fortunately, we left the "hype" phase for large data, because we have witnessed adoption and reception in almost all industries. Like the industrial revolution, large data will continue to bring technology revolution in various forms. All "smart" features that appear in the current product are based on analytics and data, which is proof that data science is the main foundation for business and technology innovation.
So, what exactly is data science? Data science is a field interdisciplinary. This is a combination of data, science, technology, and business impacts. The business value of the process is very important and usually employs advanced tools and techniques to extract the knowledge and insights that can be followed up from structured or unstructured data to optimize business goals.
Wikipedia defines it as a "field of scientific methods, processes, algorithms, and systems to extract knowledge or insight from data in various forms, both structured or unstructured, such as data mining." While the definition of data science is widely accepted, the implications and implementation of IT in the real world remain a little mystery. To go down to business implications, we need to better understand the main building blocks of science and how they are tied together. In this article, I will summarize our discussion around four main components of data science: data, science, technology, and business.
Data
Data is the most important component in data science. The important thing is not the size of the data (the term "big" is relative) but how it is used. This idea has been dubbed in a more reasonable term: smart data. While four vs are famous (volume, speed, variety, and surprises) explain the landscape of the underlying data, it is an important value in the end. Velocity makes it very difficult to maintain and analyze more than two million data per day. Feature Engineering, I.e. Creating meaningful/useful RAW data attributes is a key trend in the room. Other key trends use engineering features to handle unstructured data by instilling them in a powerful machine learning model such as inner neural networks.
Science
Data processing algorithm (better known as machine learning) is the backbone of data science. A data scientist follows a strict process (such as Crisp-DM) to explore and analyze datasets when training and building a machine learning model.
Machine learning models resolve certain problems such as predicting customer churn or identifying the most influential factors in the purchase pattern. Starting from neural networks in the 1950s, providing advanced algorithms such as vector machine support and random forests, machine learning has not disappointed practitioners. The most interesting is direct feedback from the model through the train testing process. If done correctly, there is always added value from this exploration even when the final model does not reach the desired goal.
Technology
Progress in data processing and management tools has placed life into a machine learning model. While conventional spreadsheets and SQL continue to be the main tool, there has been an incredible number of tools that recently entered the landscape, especially when scale and rapid development are a choice. Who would think a few years ago that Python and NoSQL each will compete with Java and SQL, respectively? We have seen rapid progress and adoption of open-source tools, cloud platforms, Saas, and fire.
Business
KPI Business and its impact are the most important aspect and are underestimated among many newcomers to data science. Now and then, I met with a fan of data science, new graduates, and researchers with bright eyes (I used to have such a partner) who believed that being a data scientist meant defeating several benchmarks. Not! It's about meeting several purposes: business objectives in 99% of cases. Yes, there are cases and situations where you will be challenged by the underlying problem and must show a miracle, but it's not the starting point.
Most traditional businesses are in the transition phase, even in the digitization phase, so many problems can be resolved through automation, data analysis, and predictive modeling. In my short career, I have witnessed a success story in various applications: estimated volume, churn prediction, routing optimization, real-time offer, fine wheat image recognition, plant optimization, web analysis, insurance estimation, and vehicle control optimization, for some names.
Also read about:
Why Should You outsource Your Project with App Developers India
The importance of choosing the correct winter sports gear
5 Types of Modern Office Furniture Must Haves